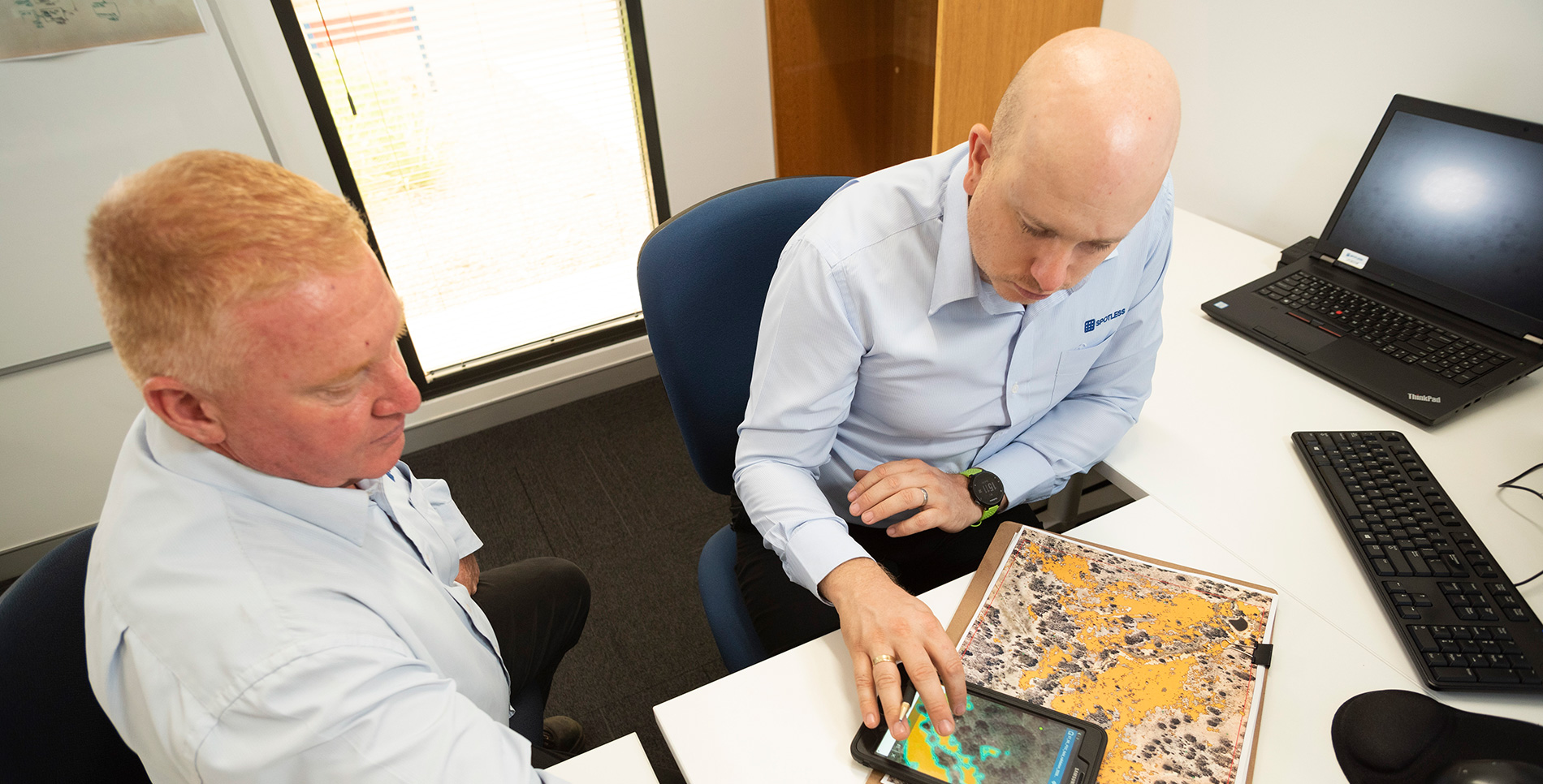
Case study
Spotless uses machine learning to knock out noxious weeds
Spotless contributes to the effective delivery, operation and maintenance of Australian Defence Estates across 380 properties and more than 2.7 million hectares of land.
Among the services we provide is land management, which includes regular field surveys to inspect and manage abundant and invasive weed species, flora and fauna and bushfire threats.
These field surveys are often required in remote and rural areas, under harsh environmental conditions and conducted over several weeks, exposing land managers to many field hazards such as uneven terrain, venomous animals and ultra violet radiation.
In July 2019, Spotless investigated innovative solutions to conduct these inspections in a way that would be safer for both our people and the environment.
Spotless partnered with the Downer Defence Data Science team to explore the use of machine learning as a land surveying data capture tool. Machine learning is an application of artificial intelligence (AI) using algorithms to self-learn from data. Through training, a machine learning model can identify a specific target from imagery.
Spotless undertook a Proof of Concept (PoC) pilot to determine if machine learning could be used to effectively identify a target weed species from commercially available imagery and use that data to develop and implement a management program. A host site was chosen for its known prevalence of serrated tussock – a declared noxious weed that can have adverse environmental effects if not managed.
The pilot was conducted over a 16-week period, the machine learning model was trained with detailed survey data and validated through field inspections to confirm the model results.
A sample plot area with known areas of serrated tussock from previous manual field surveys was marked out. Aerial imagery was then fed into the machine learning model and object detection algorithms were applied to train the machine intelligence to recognise the weed.
Based on the PoC data, the pilot recorded 85 per cent accuracy identifying tussock within the trial area, demonstrating that the approach can provide an effective level of detail and accuracy to support data-driven recommendations for land management, in particular noxious weeds and native flora management.
The machine learning model can deliver a range of benefits, including significant reduction in the time taken to obtain weed data, mitigation of weather or accessibility issues, improvement to employee safety, and no disruption to Defence capability.
It also delivers significant GHG emissions reduction and cost savings to our customer. Conducting land management activities and surveys on terrain of this size costs Defence an estimated $40,000 per inspection – equating to approximately $685,000 per annum. While there is an initial investment required to set up the technology, the capital investment is offset by removing the need for physical land surveys, providing an attractive return on investment.
This initiative demonstrates Downer’s contribution to achieve the following Sustainable Development Goals: #3 Good health and wellbeing; #9 Industry, innovation and infrastructure; #13 Climate action; and #15 Life on land
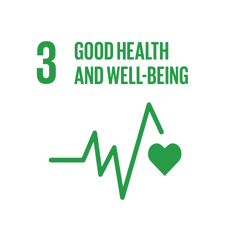
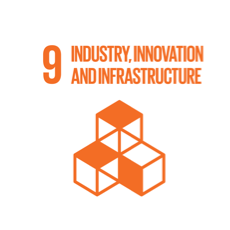
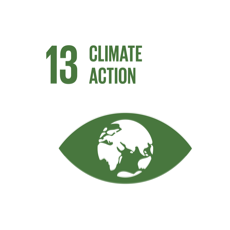
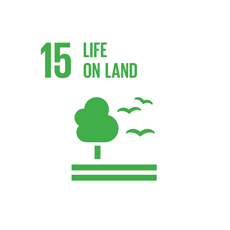